7 Costly Mistakes Companies Make When Adopting AI - and How to Avoid Them
Learn the top mistakes companies make when adopting AI and how to avoid them for successful implementation and long-term growth.
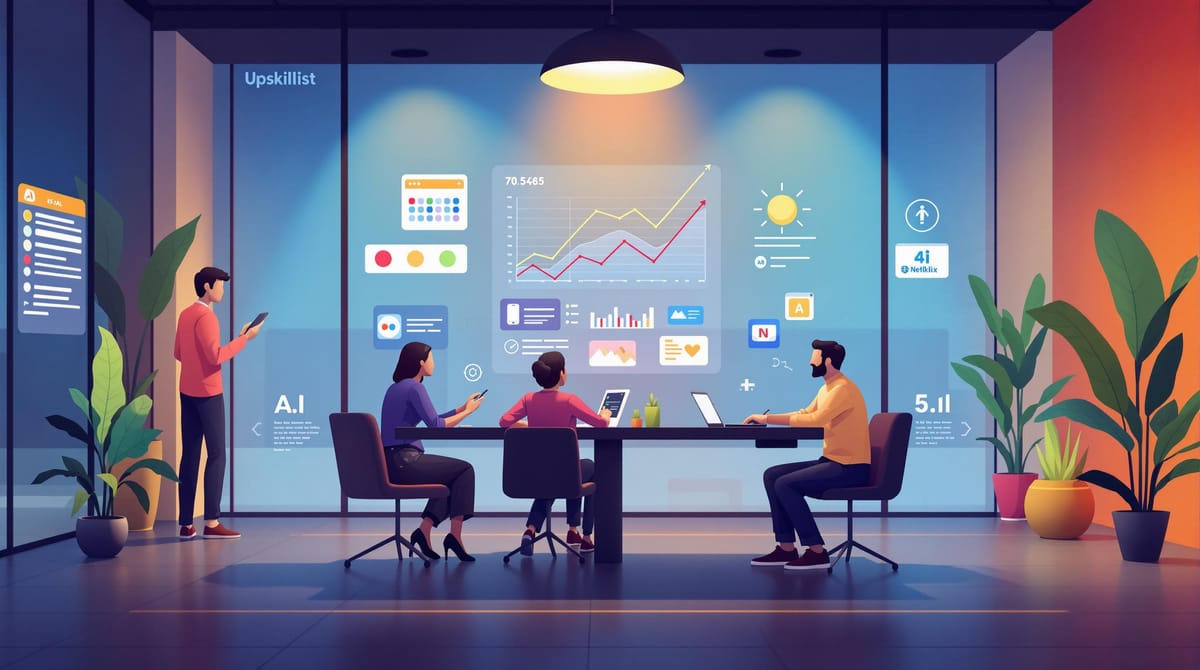
AI can transform businesses, but diving in without a plan often leads to wasted time, money, and effort. Here’s a quick summary of the most common mistakes and how to avoid them:
- Undefined Goals: Without clear objectives, AI projects fail. Define measurable goals upfront.
- Employee Resistance: Fear of job loss hinders adoption. Involve employees early, communicate benefits, and offer training.
- Lack of Training: Untrained teams make errors. Invest in expert-led training tailored to roles.
- Poor Data Practices: Bad data leads to bad results. Standardize, validate, and update your data regularly.
- Over-Reliance on AI: AI isn’t perfect. Balance AI with human judgment for better decisions.
- Ignoring Ethics: Neglecting ethical considerations risks legal trouble. Focus on privacy, bias, and transparency.
- No Scaling Plans: Many stop after initial success. Plan for growth from the start with scalable systems.
Key Takeaway: Success with AI requires clear goals, strong data, employee buy-in, and careful planning. Avoid these pitfalls to maximize AI’s potential for your business.
7 Major AI Implementation Mistakes
1. Undefined Project Goals
Diving into AI without clear, measurable goals can lead to wasted efforts and misaligned priorities. A well-thought-out strategy with specific objectives ensures resources are used effectively. Once goals are set, aligning your team with these objectives becomes the next critical step.
2. Employee Pushback
Resistance from employees can slow down AI adoption. A common fear is job loss, which can reduce cooperation and stall progress.
To overcome this, organizations should:
- Involve employees early: Include them in the planning stages to build trust.
- Communicate clearly: Emphasize how AI will support their roles, not replace them.
- Offer training: Provide targeted learning opportunities to help employees adapt.
3. Insufficient Training
When employees lack proper training, productivity drops, and mistakes increase. To avoid this, focus on expert-led training programs that cover:
- Practical use of AI tools
- Understanding AI’s strengths and limitations
- Seamlessly integrating AI into current workflows
4. Poor Data Practices
AI systems are only as good as the data they process. Low-quality data can undermine even the best AI projects. To ensure success, organizations must prioritize proper data management.
Data Issue | Impact | Solution |
---|---|---|
Inconsistent formats | Leads to inaccurate results | Standardize data inputs |
Missing values | Creates incomplete insights | Enforce data validation |
Outdated information | Results in poor decisions | Regularly update datasets |
5. Over-Reliance on AI
Leaning too heavily on AI can diminish critical thinking and lead to missed human insights. Striking the right balance between automation and human input is essential.
Human oversight should remain a priority in areas like:
- Strategic decisions
- Managing customer relationships
- Tackling complex, creative challenges
6. Ignoring Ethics and Compliance
Neglecting ethical guidelines and regulatory requirements can lead to legal and reputational risks. A framework for responsible AI use is non-negotiable.
Focus on:
- Protecting data privacy
- Preventing algorithmic bias
- Ensuring transparency in AI-driven decisions
These steps not only mitigate risks but also build trust with stakeholders.
7. Lack of Scaling Plans
Many companies fail to consider how to grow their AI initiatives after initial success. Planning for scalability requires attention to infrastructure, resource allocation, and seamless integration.
Avoiding these mistakes starts with proactive planning. Comprehensive workshops can help businesses turn their AI ambitions into actionable strategies, addressing these challenges head-on and setting the stage for long-term success.
How to Fix Common AI Mistakes
Steps for Success
Achieving success with AI requires setting clear goals, tracking measurable outcomes, and maintaining strong data practices. This includes validating, standardizing, and regularly updating your data.
To address resistance from employees, focus on open communication, offer thorough training, and create cross-functional teams that bring together experts and end-users. This collaborative approach encourages smoother adoption.
Key Implementation Steps:
1. Define Clear Success Metrics
Set measurable goals and track key performance indicators (KPIs) such as:
- Improvements in process efficiency
- Reduced operational costs
- Better quality outcomes
- Higher customer satisfaction scores
2. Strengthen Your Data Practices
Ensure your data is reliable by:
- Conducting regular audits
- Using automated validation tools
- Standardizing how data is collected
- Setting up systems for continuous monitoring
3. Invest in Training Programs
Develop training paths tailored to different roles and skill levels. Focus on how AI tools can be applied to everyday tasks for maximum impact.
The following table outlines common challenges and practical solutions to address them.
Problem-Solution Guide
Challenge | Risk Level | Solution Strategy | Expected Outcome |
---|---|---|---|
Unclear Objectives | High | Define measurable KPIs and success metrics | Better focus and improved ROI |
Data Quality Issues | Critical | Use automated data validation systems | More accurate AI models |
Employee Resistance | High | Provide training and clear communication | Higher adoption rates |
Scaling Problems | Medium | Build modular AI systems for growth | Easier scalability |
Ethical Concerns | Critical | Create an AI governance framework | Compliance with regulations |
Training Gaps | High | Offer expert-led workshops and ongoing learning | Increased skill levels |
Over-dependence | Medium | Balance human-AI collaboration | Better resource management |
Expert Insight: Equip your teams with AI training tailored to various business needs. Be transparent about AI's purpose - it's there to support human decision-making, not replace it.
AI adoption is a continuous process. Use real-time feedback to refine your approach, monitor your data's quality, and adjust training and ethical guidelines as needed.
The Biggest Mistakes Companies are Making with AI with ...
AI Skills and Career Growth
AI expertise is becoming a key driver for career advancement. As more organizations integrate AI technologies, professionals who can effectively manage and implement these tools are seeing new opportunities for growth.
Career Impact of AI Expertise
AI skills are in demand across various business areas, with notable growth in:
- Human Resources: Streamlining recruitment and improving employee engagement.
- Marketing: Automating campaigns and refining customer segmentation.
- Operations: Enhancing workflows through automation and predictive maintenance.
- Finance: Strengthening risk management and detecting fraud.
- Customer Support: Leveraging chatbots and automating service processes.
Building Core AI Skills
To stay competitive, focus on developing these essential AI-related skills:
- Technical Foundation
A solid understanding of AI basics allows professionals to make smarter decisions about integrating AI into their work.
- Strategic Implementation
This involves mastering areas like:
- Pinpointing where AI can make the most impact.
- Creating clear implementation plans.
- Measuring returns on AI investments.
- Expanding successful AI initiatives.
- Specialized Applications
Industry-specific training ensures teams can use AI tools effectively, improving both individual and organizational performance.
These skills not only enhance your current role but also set the stage for leadership opportunities.
Impact on Leadership Roles
Professionals with the ability to guide AI adoption are increasingly stepping into leadership positions. Skills like managing digital transformation projects, making informed AI-related decisions, forming cross-functional teams, and ensuring ethical AI use are highly sought after in leaders.
Continuous Learning Framework
Building your AI expertise is a step-by-step process. Here's a breakdown of how skill levels align with career growth:
Skill Level | Focus Areas | Career Impact |
---|---|---|
Beginner | Learning AI basics and initial applications | Improved job performance |
Intermediate | Strategic planning and team management | Influence at the department level |
Advanced | Scaling AI across the organization | Opportunities at the executive level |
Ongoing education - through webinars, workshops, and specialized training - keeps professionals up to date and ready for the next step in their careers.
Next Steps for AI Success
Equipped with stronger AI skills and leadership insights, it's time to refine your approach for achieving success with AI. Start by conducting an AI maturity assessment to pinpoint any gaps in your organization's preparedness.
Create an implementation roadmap built around three core areas:
-
Team Development
Invest in focused AI training for developers, managers, and end-users. Tailor the training to specific roles to ensure practical applications and measurable outcomes. -
Expert Guidance
Work with AI experts to craft strategies, evaluate risks, optimize performance, and address ethical concerns. Stay informed about industry changes to keep your strategy relevant. -
Implementation Process
Use a phased approach: evaluate readiness, plan with clearly defined ROI goals, test with pilot projects, and expand successful initiatives.
This roadmap builds on earlier strategies like setting clear objectives, nurturing talent, and prioritizing ethical AI use. By following these steps, you can avoid common mistakes and achieve meaningful, long-term results.
Stay informed about AI advancements through webinars, workshops, and training programs. When combined with human judgment, AI can significantly improve operations. Assess your organization's readiness and create a solid training plan to fully integrate AI into your processes.